Tl;dr: Summarization.
Lynn Cherny, PyData Warsaw 2018
@arnicas
Slides:
https://ghostweather.slides.com/lynncherny/tl-dr-summarization
What does the NLP world mean by it?
Structured Data? Not usually.
- Can be processed by counting things or by getting well-formed semantic/field input.
- NLP summary is possibly a similar problem afterwards, though.

Data-to-Text Generation with Content Selection and Planning, Puduppully et al 2018
Natural Language Generation from Structured Data, Matulik 2018
Neural Text Generation from Structured Data, Facebook Research, Lebret et al. 2018
Really random recent examples of structured data...

frederick parker-rhodes ( 21 november 1914 – 2 march 1987 ) was an australian rules footballer who played with carlton in the victorian football league ( vfl ) during the XXXXs and XXXXs .
Important Overview
I : source text interpretation to source text representation
T : source representation transformation to summary text representation
G : summary text generation from summary representation.
- input:
- single doc
- multiple doc
- purpose:
- informative-purpose of an informative summary is to replace the original documents
- or "indicative" -not a replacement
- generic or user/query-oriented
- general purpose or domain specific
- output:
- extractive
- abstractive
from Sparck Jones 1999
Extractive vs. Abstractive
Extractive:
Base the summary on text in the original document(s). Means sentence representation and "scoring" for ranking purposes for retrieval.
Abstractive:
Generalize from the source text(s) and produce original text summaries. I.e., identify the main ideas in the documents and encode them into feature representations. Then pass to natural language generation (NLG) systems.
Original
Universities Minister Sam Gyimah has confirmed plans for universities to be able to charge higher fees for shorter, more intensive courses.
It would mean paying about £5,500 less than for a three-year course - which would mean about £11,000 per year.
But raising annual fees above £9,250 would require Parliamentary approval.
Extraction
Universities Minister Sam Gyimah has confirmed plans for universities to be able to charge higher fees for shorter, more intensive courses.
It would mean paying about £5,500 less than for a three-year course - which would mean about £11,000 per year.
Abstraction
Universities may be able to charge higher fees for shorter courses. But raising fees requires Parliamentary approval.
Some good recent-ish summaries of summarization issues/tech
- FastForward Labs post Progress in Text Summarization (2018)
- Yue Dong's Survey on Neural Network-Based Summarization Models (2018)
- Awesome Text Summarization (a github overview article)
- Seb Ruder's Site with Code & Paper Links
- FastML Introduction to Pointer Networks with a lot of code links
- Abigail See's post "Training RNNs for Better Summarization"
Evaluation
must be discussed....
Ideal Scoring Goals (if a human did itP
"The factors that human experts must consider when giving scores to each candidate summary are grammaticality, non-redundancy, integration of most important pieces of information, structure and coherence."
Actual Eval Metrics
Since asking a human to manually evaluate millions of summaries is long and impractical at scale, we rely on an automated evaluation metric called ROUGE (Recall-Oriented Understudy for Gisting Evaluation). ROUGE works by comparing matching sub-phrases in the generated summaries against sub-phrases in the ground truth reference summaries.
Src: Salesforce Blog
....the summaries with the highest ROUGE aren't necessarily the most readable or natural ones.
Gold Summary by Human: A good diet must have apples and bananas.
Model output: Apples and bananas are must for a good diet.
(Alternative model output): a apples bananas and good diet must
If we use the ROUGE-1, the score is 7/8 = 0.875.
Same for the alternative word salad!
For ROUGE-2, 1st model output would get 4/7 = ~0.57.
"Good" Rouge Scores Table

- They only assess content selection and do not account for other quality aspects, such as fluency, grammaticality, coherence, etc.
- To assess content selection, they rely mostly on lexical overlap, although an abstractive summary could express they same content as a reference without any lexical overlap.
- Given the subjectiveness of summarization and the correspondingly low agreement between annotators, the metrics were designed to be used with multiple reference summaries per input. However, recent datasets such as CNN/DailyMail and Gigaword provide only a single reference.
Seb Ruder's github repo of references - Warning on ROUGE Metrics in Summarization
Takeaways to be aware of
The metrics are pretty dubious.
Some of the most popular datasets are problematic (CNN/DailyMail)
There is a lot of "teaching to the test" in the academic literature.
Human review is still critical, to the degree it can be afforded.
How do we inspect the results? Often a bit visually...

abstractive
extractive
A bit on abstractive systems, the holy grail.
A general abstractive seq2seq approach

Abigail See on abstractive RNN summaries
Problem 1: The summaries sometimes reproduce factual details inaccurately (e.g. Germany beat Argentina 3-2). This is especially common for rare or out-of-vocabulary words such as 2-0.
Problem 2: The summaries sometimes repeat themselves (e.g. Germany beat Germany beat Germany beat…)

Pointer-Generator + Coverage (See et al 2017):
Encoder is a single layer bidirectional LSTM.
Decoder is a single-layer uni-directional LSTM, with a pointer for attention that copies words from the source. Plus a coverage penalty metric reduces repetition and out of vocab inaccuracies.

repetition
unknown
words,
inaccuracy
better!
Trying out some code....
But, sadly, academic code!

actual code for SummaRuNNer (Nallapati 2016, 2017)
(Audience,
I did it.)
Things that take a long time.
Is there a checkpoint available?
Is the code documented?
Do you have to build the datasets yourself?
How easy is it to retrain? Or transfer?
These are serious non-trivial questions before embarking on using someone's academic model. Budget time :)
Demo with "Hansel and Gretel" from Grimm's Fairytales
There are 2 kids, abandonned or lost in the woods... they left breadcrumb trails to get home but the birds ate them. They find a ginger-bread cottage and are welcomed by a witch. She wants to fatten them up to eat them. One of the kids shoves her in the oven and they escape!


What I Remembered (Roughly)
The real story is a little surprising... there is a ton of text "noise" in this story. (I know you can't read this...)
The kids are left TWICE in the woods, after the woodcutter's wife convinces him to do this.
"He who says A must say B, likewise, and as he had yielded the first time, he had to do so a second time also."
This weird structure happens twice:
when they had gone a little distance , hansel stood still , and peeped back at the house... " ah , father ," said hansel , " i am looking at my white cat sitting upon the roof of the house , and trying to say good - bye ." " that is not a cat ; it is only the sun shining on the white chimney ."
Gender roles: Hansel thinks of the breadcrumbs, but Grethel kills the witch and convinces a duck to save them. The step-mother and witch are evil. The father goes along with leaving them, convinced by his new wife, but remains a loved parent at the end (and she is dead).
Odd avian characters: A bird leads them to the witch's house. Birds eat the breadcrumbs. But a duck saves them going home by taking them across a stream.
More text "noise":
My tale is done .
There runs a mouse ; whoever catches her may make a great , great cap out of her fur .
--Gutenberg text of Grimm's Fairytales (maybe a different translation than mine)
"know , then , my husband , answered she , " we will lead them away , quite early in the morning , into the thickest part of the wood , and there make them a fire , and give them each a little piece of bread ; then we will go to our work , and leave them alone , so they will not find the way home again , and we shall be freed from them ."
it soon ceased , and spreading its wings flew off ; and they followed it until it arrived at a cottage , upon the roof of which it perched ; and when they went close up to it they saw that the cottage was made of bread and cakes , and the window - panes were of clear sugar .
the old woman behaved very kindly to them , but in reality she was a wicked witch who waylaid children , and built the bread - house in order to entice them in , but as soon as they were in her power she killed them , cooked and ate them , and made a great festival of the day .
" creep in , said the witch , " and see if it is hot enough , and then we will put in the bread "; but she intended when grethel got in to shut up the oven and let her bake , so that she might eat her as well as hansel .
then grethel gave her a push , so that she fell right in , and then shutting the iron door she bolted it !
this the good little bird did , and when both were happily arrived on the other side , and had gone a little way , they came to a well - known wood , which they knew the better every step they went , and at last they perceived their father ' s house .
he had not had one happy hour since he had left the children in the forest ; and his wife was dead .
My 7
line
Picks
from
Hansel
&
Gretel

Location of those lines in the text
made in R package ggpage :)
Twice left in the woods stuff -
should it be in the summary??

Using SummaRuNNer (Nallapati et al. 2016)
(compared to a lot of other code, it was somewhat easily modifiable and used a general embedding, included model checkpoints, and was easy to get the ranked sentence outputs.)
content richness,
salience,
novelty.
- The first layer of the RNN is a bi-directional GRU that runs on words level: it takes GloVe word embeddings in a sentence as the inputs and produces a set of hidden states. These hidden states are averaged into a vector, which is used as the sentence representation.
- The second layer of the RNN is also a bi-directional GRU, and it runs on the sentence-level by taking the sentence representations obtained by the first layer as inputs. The hidden states of the second layer are then combined into a document representation through a non-linear transformation.
- A sigmoid ranking function acts on the sentence reps and outputs the sorted highest scoring sentences.
Text description of the architecture of SummaRuNNer
SummaRuNNer: 3 Sample Models' Picks for Extraction. Sadness.

Observation: Top of the "article"! (Also, tbh, a cutoff is buried in the code, but that's not affecting the focus on top of the text.)
News has a structural bias.
The Inverted Pyramid:

"Abbreviations" on the training data often include chopping the documents summarized and/or sentence length to limit the number of sentences trained on.
And for the commonly used CNN/Daily Mail data:
On average, there are about 28 sentences per document in the training set, and an average of 3-4 sentences in the reference summaries. This is short stuff.
And hidden in the news data focus.... shortness.
Try online Sidenet and Refreshnet
Refresh frames extractive summarization as a sentence ranking task and uses a novel training algorithm which globally optimizes the ROUGE evaluation metric through a reinforcement learning objective.
Sidenet tries to use title and image captions to help with the summary.
Deep Problems
Kedzie, McKeown, Daumé (2018) at EMNLP
1. Sentence position dominates the signal for news, but not other genres.
2. Word embedding averaging is as good or better than RNNs/CNNs for sentence embedding.
3. Pre-trained word embeddings are as good or better than learned embeddings in 5/6 datasets.
4. Non auto-regressive sentence extraction performs as good or better than auto-regressive.
Problem with a lot of Abstractive work
However, those authors would concede what Noah Weber and collaborators showed last month: in practice, these abstractive networks work by “mostly, if not entirely, copying over phrases, sentences, and sometimes multiple consecutive sentences from an input paragraph, effectively performing extractive summarization.” So, for now at least, you get the training data requirements and engineering complexity of cutting-edge deep learning without the practical performance increase.
see also EMNLP paper - Zhang et al. 2018 ("we also implemented a pure copy system")
We find that many sophisticated features of state of the art extractive summarizers do not improve performance over simpler models. These results suggest that it is easier to create a summarizer for a new domain than previous work suggests and bring into question the benefit of deep learning models for summarization for those domains that do have massive datasets (i.e., news).
Other genres have structural assets too (requiring complete retraining, for a neural approach)

scientific papers
(with abstracts and keywords),
fiction,
product reviews,
life stories / obituaries,
emails,
tweet exchanges,
dialogue,
etc.
Non-neural approaches
NB: I highly recommend Allahyari et al for review of non-neural approaches to summarization, including topic modeling during intermediate representation step.
Early extractive work (non-neural)
[Extractive] algorithms rank each sentence based on its relation to the other sentences by using pre-defined formulas such as the sum of frequencies of significant words (Luhn algorithm [Luhn, 1958]); the overlapping rate with the document title [EEEEEEEEE!] (PyTeaser [Xu, 2004]); the correlation with salient concepts/topics (Latent Semantic Analysis [Gong and Liu, 2001]); and sum of weighted similarities to other sentences (TextRank [Mihalcea and Tarau, 2004] ).
Yue Dong review paper (Apr 2018)
Gensim's Summarize
- Pre-process the text: remove stop words and stem the remaining words.
- Create a graph where vertices are sentences.
- Connect every sentence to every other sentence by an edge. The weight of the edge is how similar the two sentences are.
- Run the PageRank algorithm on the graph.
- Pick the vertices (sentences) with the highest PageRank score.
based on TextRank alg too.


- " know , then , my husband ," answered she , " we will lead them away , quite early in the morning , into the thickest part of the wood , and there make them a fire , and give them each a little piece of bread ; then we will go to our work , and leave them alone , so they will not find the way home again , and we shall be freed from them ."
- the children , however , had heard the conversation as they lay awake , and as soon as the old people went to sleep hansel got up , intending to pick up some pebbles as before ; but the wife had locked the door , so that he could not get out .
- then they went to sleep ; but the evening arrived and no one came to visit the poor children , and in the dark night they awoke , and hansel comforted his sister by saying , " only wait , grethel , till the moon comes out , then we shall see the crumbs of bread which i have dropped , and they will show us the way home ."
- hansel kept saying to grethel , " we will soon find the way "; but they did not , and they walked the whole night long and the next day , but still they did not come out of the wood ; and they got so hungry , for they had nothing to eat but the berries which they found upon the bushes .
- it was now the third morning since they had left their father ' s house , and they still walked on ; but they only got deeper and deeper into the wood , and hansel saw that if help did not come very soon they would die of hunger .
- hansel and grethel were so frightened that they let fall what they had in their hands ; but the old woman , nodding her head , said , " ah , you dear children , what has brought you here ?
- when hansel and grethel came near the witch ' s house she laughed wickedly , saying , " here come two who shall not escape me ."
- grethel came next , and , shaking her till she awoke , the witch said , " get up , you lazy thing , and fetch some water to cook something good for your brother , who must remain in that stall and get fat ; when he is fat enough i shall eat him ."
Gensim's TextRank Summarization
at least
the witch appears!
(we set up the final
drama.)
Sumy Python Lib
- Luhn - heuristic method, reference
- Edmundson heurestic method, reference
- Latent Semantic Analysis (LSA)
- LexRank - Unsupervised approach inspired by algorithms PageRank and HITS, reference (penalizes repetition more than TextRank, uses IDF-modified cosine)
- TextRank - Unsupervised approach, also using PageRank algorithm, reference (see Gensim above)
- SumBasic - Method that is often used as a baseline in the literature. Read about SumBasic
- KL-Sum - Method that greedily adds sentences to a summary so long as it decreases the KL Divergence. Read about KL-Sum
- hansel and grethel
- once upon a time there dwelt near a large wood a poor woodcutter , with his wife and two children by his former marriage , a little boy called hansel , and a girl named grethel .
- when they had gone a little distance , hansel stood still , and peeped back at the house ; and this he repeated several times , till his father said , " hansel , what are you peeping at , and why do you lag behind ?
- they knocked at the door , and when the wife opened it , and saw hansel and grethel , she exclaimed , " you wicked children !
- then they went to sleep ; but the evening arrived and no one came to visit the poor children , and in the dark night they awoke , and hansel comforted his sister by saying , " only wait , grethel , till the moon comes out , then we shall see the crumbs of bread which i have dropped , and they will show us the way home ."
- hansel kept saying to grethel , " we will soon find the way "; but they did not , and they walked the whole night long and the next day , but still they did not come out of the wood ; and they got so hungry , for they had nothing to eat but the berries which they found upon the bushes .
- "creep in ," said the witch , " and see if it is hot enough , and then we will put in the bread "; but she intended when grethel got in to shut up the oven and let her bake , so that she might eat her as well as hansel .
- now she ran to hansel , and , opening his door , called out , " hansel , we are saved ; the old witch is dead !"
Sumy lib's TextRank

better
spread than
gensim,
last 2 choices
are great.
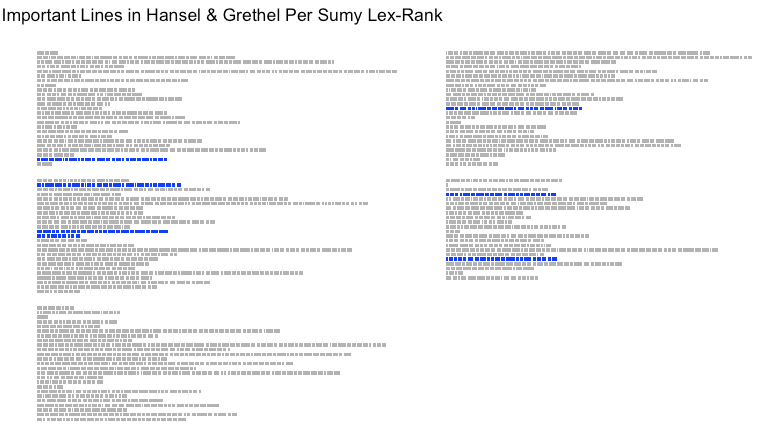
- " ah , father ," said hansel , " i am looking at my white cat sitting upon the roof of the house , and trying to say good - bye ."
- but in reality hansel was not looking at a cat ; but every time he stopped , he dropped a pebble out of his pocket upon the path .
- they knocked at the door , and when the wife opened it , and saw hansel and grethel , she exclaimed , " you wicked children !
- why did you sleep so long in the wood ?
- " grethel ," she called out in a passion , " get some water quickly ; be hansel fat or lean , this morning i will kill and cook him ."
- now she ran to hansel , and , opening his door , called out , " hansel , we are saved ; the old witch is dead !"
- he had not had one happy hour since he had left the children in the forest ; and his wife was dead .
Lines from
Sumy Lib LexRank
shorter
lines,
good spread,
conclusion
included.
Online Summarization Tool Demos
smmry.com is the most fun.
Autosummarizer is not too bad, but more opaque.
Even more broadly... what kinds of summaries are there?
Aside from news.
Useful Types To Think About
- Books (avoid spoilers - "indicative summary")
- Query-focused summaries : "What happens to the grandmother in the book/movie."
- Content-based recommender systems (cold-start problem, e.g., Spotify's Release Radar)
- TV & Movies (trailers & reviews)
- Product reviews - judgment + description
- Garbage book summaries for school kids and managers (Cliffs Notes, condensed biz books)
Skip these in the talk,
sorry.
Recommender Systems : Content (and social) relationships
Do they use content analysis? Some do... and some do very little:

Since the output of the algorithm is content that is relatively new, the algorithm cannot rely purely on user data, and instead opts to make use of the complex data embedded in the very timbers, tempos, and sounds of the newly released tracks. This allows Spotify to deliver intelligent curation of content, even in the absence of user data, thus resulting in music recommendations that are as accurate as technology currently allows for – by combing through literally every piece of the music.
Spotify's Release Radar - New Music Recommendation, Content-Based Analysis.

Summarizing Opinion in Product Reviews
Angelidis and Lapata 2018 paper

sentiment +!
Book reviews/blurbs by genre
The feel-good book of the year, Night of Miracles is a delightful novel of friendship, community, and the way small acts of kindness can change your life.
Abandoned is the second book in a thrilling sci-fi action adventure set on Donovan, a treacherous alien planet where corporate threats and dangerous creatures imperil the lives of the planet’s colonists.
from the Penguin site




Creative Extraction
Extractive text as art seed,
commentary,
conversation.


Grahame-Smith began with the original text of Austen's novel, adding zombie and ninja elements while developing an overall plot line for the new material; "you kill somebody off in Chapter 7, it has repercussions in Chapter 56". According to the author, the original text of the novel was well-suited for use as a zombie horror story:
You have this fiercely independent heroine, you have this dashing heroic gentleman, you have a militia camped out for seemingly no reason whatsoever nearby, and people are always walking here and there and taking carriage rides here and there ... It was just ripe for gore and senseless violence. From my perspective anyway.
See also several #Nanogenmo projects, including Martin O'Leary's Mashups
Text
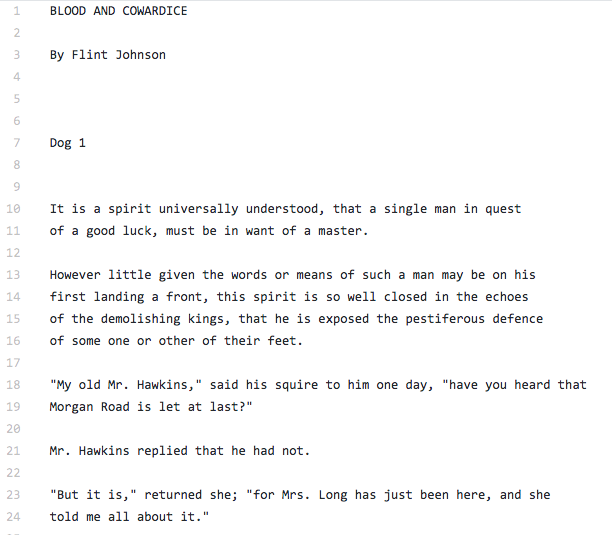
Pride and Prejudice and Treasure Island:

Found poetry is a type of poetry created by taking words, phrases, and sometimes whole passages from other sources and reframing them as poetry
- Take, as input, an image of text, from a newspaper or book.
- Run OCR against the image, identifying the words and their bounding boxes.
- Feed the extracted text into a natural language parser, categorizing each part of speech.
- Given one of many randomly selected Tracery grammars, select words from the current page that match the parts of speech of that grammar.
- Draw around those words and "scribble" out all other text on the page image.
- Output the final page as a new image.
Trailers are extractive summaries!
Even More Creative Film and TV Remixes
Of Oz the Wizard is another highly conceptual remix that alphabetises and re-orders every line of dialogue from The Wizard of Oz (1939). Created by coder Matt Bucy as the result of a challenge posed by a friend, it was partly achieved by using an app to isolate each line. As Bucy said in one interview: “Basically, it was edited in Excel.”
Fan Edits (and other remixes)
Star Wars: The Phantom Edit
- Opening crawl replaced with a new one explaining why the edit was made
- Re-editing of nearly all scenes featuring Jar Jar Binks and removing some of what Nichols dubs 'Jar Jar Antics'
- Removal or re-editing of most of the Battle Droid dialogue
- Limiting of exposition throughout the film
- Trimming scenes involving politics
- Re-arrangement of shots and scenes to match the original Star Wars trilogy's presentation style
- Removal of "Yippee" and "Oops" from Anakin's dialogue
- Removal of dialogue that specifies the nature of midi-chlorians as a biological basis for Force sensitivity
- Reinstatement of deleted scenes in order to fill in plot holes in the film narrative
"Slash" videos use tv show excerpts in a music video format, to re-interpret show relationships viewed thru a queer eye. They've been made (mostly by women) for decades, even before digital editing was a thing.
(This is a pretty hilarious intro.)
Artists (and interesting researchers) should ask the extreme questions...
Can you summarize the English language?
The Bible or Koran?
Wikipedia?
What happens if you summarize a poem?
can you summarize a haiku?

The "heresy of paraphrase"
[Cleanth] Brooks argued that meaning in poetry is irreducible, because "a true poem is a simulacrum of reality...an experience rather than any mere statement about experience or any mere abstraction from experience."
Thanks to Ted Underwood for pointing me to this.
A poem should be palpable and mute
As a globed fruit
Dumb
As old medallions to the thumb
Silent as the sleeve-worn stone
Of casement ledges where the moss has grown –
A poem should be wordless
As the flight of birds
A poem should be motionless in time
As the moon climbs
Leaving, as the moon releases
Twig by twig the night-entangled trees,
Leaving, as the moon behind the winter leaves,
Memory by memory the mind –
A poem should be motionless in time
As the moon climbs
A poem should be equal to:
Not true
For all the history of grief
An empty doorway and a maple leaf
For love
The leaning grasses and two lights above the sea –
A poem should not mean
But be.
Ars Poetica, by Archibald MacLeish

How do we decide what's important when we summarize?
Even for the same goal, we have different eyes.
Image captioning
(definitely abstractive)


Family photo. You should read my piece on
COCO's memory palace, it's fun.

Improving Abstraction in Text Summarization, Krys ́cin ́ski et al., EMNLP 2018
Let's look closely at the reference summary vs. the original article....
?
Quoting Out of Context
The credit sequence, with its jumpy frames and near-subliminal flashes of psychoparaphernalia, is a small masterpiece of dementia
a small masterpiece
Marya McQuirter, a historian at the Smithsonian Institution’s National Museum of African American History and Culture, recalls searching the Smithsonian’s internal catalog for the terms "black" and "white.” Searching the millions of catalog entries for “black” yielded a rich array of objects related to Black people, Black culture, and Black history in the US.... But searching for “white” yielded only white-colored visual art. Almost nothing showed up relating to the history of white people in the United States.
McQuirter, who is Black, knew the reason why: in the United States, it’s white people and their bodies who occupy the “default” position.
-Data Feminism, by Catherine D'Ignazio and Lauren Klein
Things that are not there can't be summarized.
We can't afford to not worry about bias anymore.
We can tell new, shorter stories with summaries and extraction - but we can also distort or lose the point of the original.
thank you!
arnicas@gmail.com
@arnicas
Slides:
https://ghostweather.slides.com/lynncherny/tl-dr-summarization
Jobs
My client SpiritAI: Working on hate speech detection and character dialogue modeling. Remote consulting available.
Also need Spanish language and Russian language NLP help.
Talk to me if you want more info.
Tl;dr: Summarization.
By Lynn Cherny
Tl;dr: Summarization.
A talk overviewing NLP summarization goals and metrics, given as keynote at PyData Warsaw, with some non-news experiments and commentary on artistic applications.
- 5,590